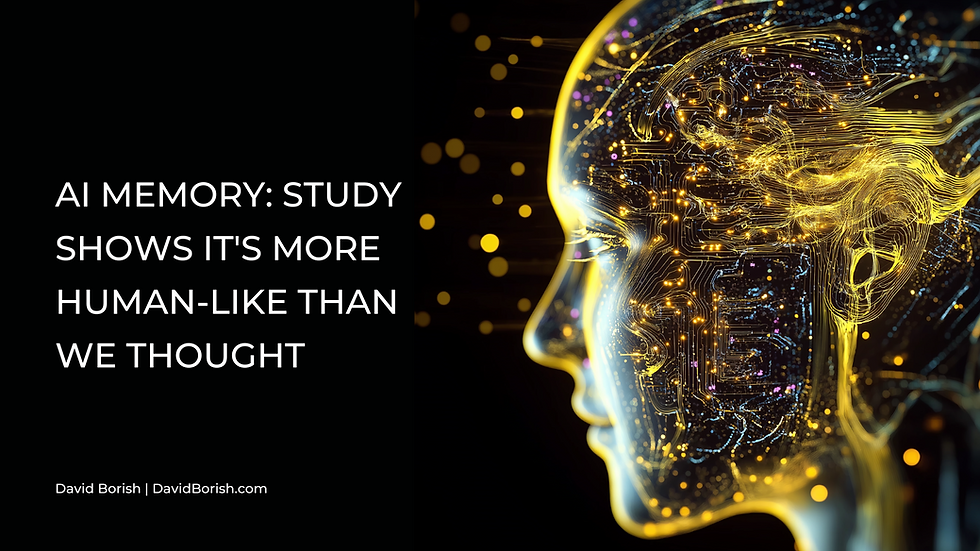
Recent research from Hong Kong Polytechnic University has unveiled striking similarities between the memory capabilities of large language models (LLMs) and human cognition. The study, titled "Schrödinger's Memory: Large Language Models," challenges our understanding of artificial intelligence and its cognitive processes.
The researchers, led by Wei Wang and Qing Li, propose that LLMs possess a form of memory they call "Schrödinger's memory." This concept suggests that an LLM's memory only becomes observable when queried, much like how humans can only verify their memories by answering specific questions.
To test this theory, the team conducted experiments using various LLMs, including Qwen and Bloom models. They trained these models on datasets of Chinese and English poetry, then tested their ability to recall entire poems based on minimal input information, such as the title and author.
The results were remarkable. Some models demonstrated near-perfect recall, remembering up to 99.9% of the poems they were trained on. This performance far exceeded what an average human could achieve under similar conditions.
However, the researchers argue that this isn't simple data storage and retrieval. Instead, they propose that LLMs dynamically generate responses based on input, similar to how humans reconstruct memories. This aligns with the Universal Approximation Theorem (UAT), which the authors use to explain the underlying mechanisms of LLM memory.
The study also found that longer input texts were harder for LLMs to remember accurately, mirroring human memory limitations. This further supports the idea that AI memory processes may be more analogous to human cognition than previously thought.
The implications of this research are profound. It suggests that the gap between artificial and human intelligence may be narrower than we imagined, at least in terms of memory processes. As we continue to develop more advanced AI systems, understanding these similarities could lead to more effective and human-like AI interactions.
Potential future impacts of this discovery:
Enhanced AI interactions: Understanding the similarities between AI and human memory could lead to more natural and intuitive human-AI interactions.
Improved AI training methods: Insights into how LLMs process and recall information could inform new, more efficient training techniques.
Advancements in cognitive science: The parallels between AI and human memory may provide new avenues for studying human cognition and neuroscience.
Ethical considerations: As AI systems become more human-like in their cognitive processes, it may raise new ethical questions about AI rights and responsibilities.
Personalized AI assistants: With a better understanding of AI memory, we could develop more personalized and context-aware AI assistants that can maintain long-term "relationships" with users.
Educational applications: AI systems with human-like memory could be leveraged to create more effective and adaptive learning tools.
Healthcare innovations: In fields like mental health, AI with human-like memory processes could potentially assist in diagnosis or treatment of memory-related disorders.
As we continue to unravel the mysteries of artificial intelligence, discoveries like this remind us that the line between human and machine cognition may be blurrier than we once thought. The future of AI, informed by these insights, promises to be both exciting and profoundly impactful across numerous fields of human endeavor.
Comments