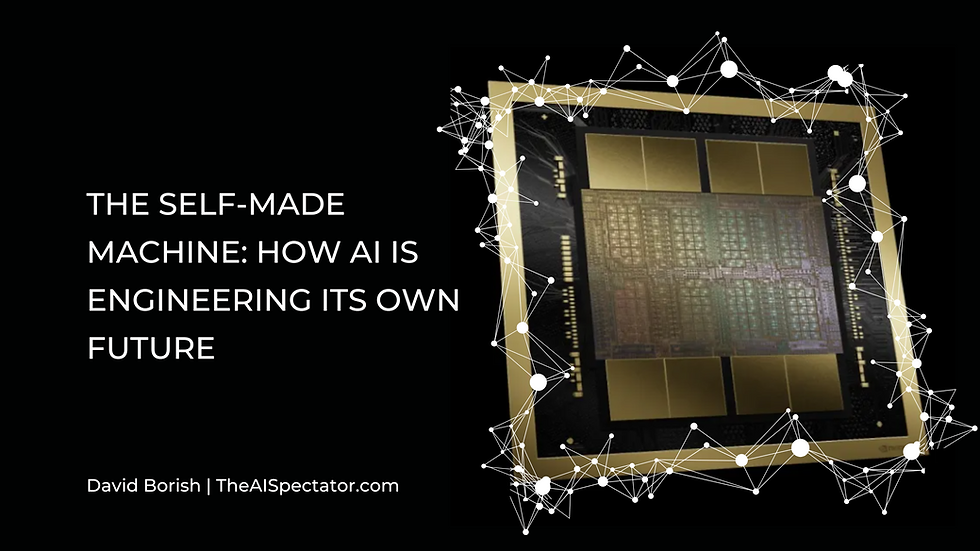
In a remarkable display of technological recursion, artificial intelligence has begun to play a crucial role in designing the very hardware that powers it. This self-reinforcing cycle represents one of the most significant developments in computing history, where AI systems are actively participating in their own evolution.
The Foundation: AI in Chip Design
The most immediate example of AI's self-improvement capabilities lies in microchip design. Companies like Synopsys and Cadence are leveraging AI systems to optimize chip layouts in ways that human engineers never could. These AI-designed chips, in turn, power newer and more capable AI systems, creating a powerful feedback loop of technological advancement.
Through reinforcement learning, AI systems can explore design possibilities that would take human engineers lifetimes to consider. As noted by Nvidia's chief scientist Bill Dally, these systems often discover "bizarre ideas that work because they operate completely outside the way humans think."
Beyond Hardware: AI's Self-Enhancement Cascade
The self-improvement cycle extends beyond chip design. Here are other key areas where AI is contributing to its own advancement:
Neural Architecture Search (NAS) AI systems now actively participate in designing optimal neural network architectures This automation accelerates the discovery of more efficient AI models
Training Optimization AI systems help optimize training procedures for other AI models They can predict which hyperparameters will yield the best results
Code Generation AI coding assistants are helping develop better AI frameworks This accelerates the development of new AI technologies
Resource Optimization AI systems manage data center resources, improving the efficiency of AI training They optimize power consumption and computing resource allocation
The Future of Self-Improving AI
Looking ahead, we're witnessing an unprecedented acceleration in AI's self-improvement cycle. The emergence of automated ML operations represents a significant shift, where AI systems now oversee the entire machine learning lifecycle, from data preparation to model deployment and monitoring. These systems continuously optimize their processes, learning from each deployment to enhance future operations.
In parallel, we're seeing the rise of self-evolving models that can automatically update and improve their own architectures. Unlike traditional AI systems that require manual updates, these advanced models can identify areas for improvement and implement changes autonomously, leading to more efficient and capable systems over time.
The integration of hardware and software optimization marks another significant advancement. AI systems are now capable of simultaneously fine-tuning both components, creating a symbiotic relationship that ensures optimal performance at every level. This holistic approach to optimization is particularly important as we push the boundaries of what's possible with current technology.
Energy efficiency has become a central focus in this evolution, with AI systems specifically designed to create more sustainable versions of themselves. These systems are actively working to reduce their environmental impact while maintaining or improving their performance capabilities.
The Democratization Effect
The impact of these self-improving AI systems extends far beyond technical capabilities – it's fundamentally transforming who can participate in AI innovation. What was once the exclusive domain of tech giants with billion-dollar budgets is now becoming accessible to smaller teams and startups. As noted by Stelios Diamantidis from Synopsys, we're entering an era where "five folks that got together with a great idea" can design custom AI chips.
This democratization is creating ripple effects throughout the technology sector. Small teams can now compete with larger corporations in specialized AI applications. Universities and research institutions with limited resources can contribute meaningful innovations to the field. Startups can develop custom AI solutions without massive initial investments in infrastructure and expertise.
The implications of this accessibility are profound. Innovation is no longer limited by organizational size or budget but rather by creativity and vision. We're seeing the emergence of specialized AI solutions for niche markets that larger companies might have overlooked. From AI-powered agricultural solutions in developing regions to custom medical diagnosis tools for rare conditions, these smaller teams are addressing specific needs with unprecedented precision and efficiency.
The Path Forward
As AI continues to participate in its own development, we're likely to see accelerating progress in both hardware and software capabilities. This self-reinforcing cycle could lead to more efficient, powerful, and accessible AI systems, potentially transforming how we approach technological innovation.
The key challenge moving forward will be ensuring this self-improvement cycle remains aligned with human needs and values while maintaining its impressive pace of innovation.
Comments